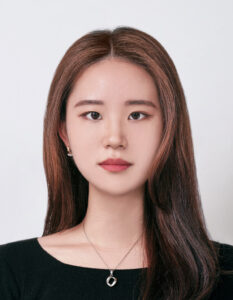
유미리
Miri Yu
zztiok at ajou.ac.kr
Research interests
Cloud Computing, Distributed System, Federated Learning
Introduction
Miri Yu is a master’s course student of the Department of Artificial Intelligence of Ajou University
Publications
2024
Yu, Miri; Choi, Jiheon; Lee, Jaehyun; Oh, Sangyoon
Staleness Aware Semi-asynchronous Federated Learning Journal Article
In: Journal of Parallel and Distributed Computing, 2024.
@article{miri2024staleness,
title = {Staleness Aware Semi-asynchronous Federated Learning},
author = {Miri Yu and Jiheon Choi and Jaehyun Lee and Sangyoon Oh},
url = {https://www.sciencedirect.com/science/article/pii/S074373152400114X},
year = {2024},
date = {2024-07-01},
urldate = {2024-07-01},
journal = {Journal of Parallel and Distributed Computing},
abstract = {As the attempts to distribute deep learning using personal data have increased, the importance of federated learning (FL) has also increased. Attempts have been made to overcome the core challenges of federated learning (i.e., statistical and system heterogeneity) using synchronous or asynchronous protocols. However, stragglers reduce training efficiency in terms of latency and accuracy in each protocol, respectively. To solve straggler issues, a semi-asynchronous protocol that combines the two protocols can be applied to FL; however, effectively handling the staleness of the local model is a difficult problem. We proposed SASAFL to solve the training inefficiency caused by staleness in semi-asynchronous FL. SASAFL enables stable training by considering the quality of the global model to synchronise the servers and clients. In addition, it achieves high accuracy and low latency by adjusting the number of participating clients in response to changes in global loss and immediately processing clients that did not to participate in the previous round. An evaluation was conducted under various conditions to verify the effectiveness of the SASAFL. SASAFL achieved 19.69%p higher accuracy than the baseline, 2.32 times higher round-to-accuracy and 2.24 times higher latency-to-accuracy. Additionally, SASAFL always achieved target accuracy that the baseline can't reach.},
keywords = {},
pubstate = {published},
tppubtype = {article}
}
2023
Yu, Miri; Kwon, Oh-Kyoung; Oh, Sangyoon (Ed.)
Addressing Client Heterogeneity in Synchronous Federated Learning: The CHAFL Approach Conference
The 29th IEEE International Conference on Parallel and Distributed Systems (ICPADS 2023), 2023.
@conference{nokey,
title = {Addressing Client Heterogeneity in Synchronous Federated Learning: The CHAFL Approach},
editor = {Miri Yu and Oh-Kyoung Kwon and Sangyoon Oh},
year = {2023},
date = {2023-11-10},
urldate = {2023-11-10},
booktitle = {The 29th IEEE International Conference on Parallel and Distributed Systems (ICPADS 2023)},
keywords = {},
pubstate = {published},
tppubtype = {conference}
}
Lee, Seungjun; Yu, Miri; Yoon, Daegun; Oh, Sangyoon
Can hierarchical client clustering mitigate the data heterogeneity effect in federated learning? Conference
2023 IEEE International Parallel and Distributed Processing Symposium Workshops (IPDPSW), 2023, ISBN: 979-8-3503-1200-3.
@conference{nokey,
title = {Can hierarchical client clustering mitigate the data heterogeneity effect in federated learning?},
author = {Seungjun Lee and Miri Yu and Daegun Yoon and Sangyoon Oh},
url = {10.1109/IPDPSW59300.2023.00134},
doi = {10.1109/IPDPSW59300.2023.00134},
isbn = {979-8-3503-1200-3},
year = {2023},
date = {2023-05-15},
urldate = {2023-05-15},
booktitle = {2023 IEEE International Parallel and Distributed Processing Symposium Workshops (IPDPSW)},
abstract = {Federated learning (FL) was proposed for training a deep neural network model using millions of user data. The technique has attracted considerable attention owing to its privacy-preserving characteristic. However, two major challenges exist. The first is the limitation of simultaneously participating clients. If the number of clients increases, the single parameter server easily becomes a bottleneck and is prone to have stragglers. The second is data heterogeneity, which adversely affects the accuracy of the global model. Because data should remain at user devices to preserve privacy, we cannot use data shuffling, which is used to homogenize training data in traditional distributed deep learning. We propose a client clustering and model aggregation method, CCFed, to increase the number of simultaneously participating clients and mitigate the data heterogeneity problem. CCFed improves the learning performance using set partition modeling to let data be evenly distributed between clusters and mitigate the effect of a non-IID environment. Experiments show that we can achieve a 2.7-14% higher accuracy using CCFed compared with FedAvg, where CCFed requires approximately 50% less number of rounds compared with FedAvg training on benchmark datasets.},
keywords = {},
pubstate = {published},
tppubtype = {conference}
}
2021
Yu, Miri; Lee, Seungjun; Oh, Sangyoon
Energy-aware container migration scheme in edge computing for fault-tolerant fire-disaster response system Conference
The 7th International Conference on Next Generation Computing 2021, 2021.
@conference{Yu2021container,
title = {Energy-aware container migration scheme in edge computing for fault-tolerant fire-disaster response system},
author = {Miri Yu and Seungjun Lee and Sangyoon Oh},
year = {2021},
date = {2021-11-05},
urldate = {2021-11-05},
booktitle = {The 7th International Conference on Next Generation Computing 2021},
abstract = {In light of the recent advancements made in IT, many researchers are studying and exploring ways to minimize damage from fire disasters using artificial intelligence and cloud technology. With the introduction of edge computing, fire-disaster response software systems have made significant progress. However, existing studies often do not consider the response to a sudden power supply cut-off due to fire. In this study, we propose a container migration scheme based on the first-fit-decreasing algorithm of bin-packing problem and 0-1 knapsack algorithm to provide fault tolerance for containers running on edge servers that are powered off.},
keywords = {},
pubstate = {published},
tppubtype = {conference}
}