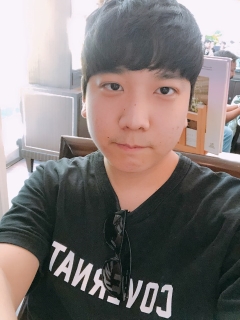
박주원
Juwon Park
pjw930731 at naver.com
After graduation
삼성전자
Publications
2022
3.
Park, Juwon; Yoon, Daegun; Yeo, Sangho; Oh, Sangyoon
AMBLE: Adjusting Mini-Batch and Local Epoch for Federated Learning with Heterogeneous Devices🌏 InternationalJournal Article
In: Journal of Parallel and Distributed Computing, 2022, ISSN: 0743-7315.
@article{Juwon2022AMBLE,
title = {AMBLE: Adjusting Mini-Batch and Local Epoch for Federated Learning with Heterogeneous Devices},
author = {Juwon Park and Daegun Yoon and Sangho Yeo and Sangyoon Oh},
url = {https://www.sciencedirect.com/science/article/pii/S0743731522001757},
doi = {https://doi.org/10.1016/j.jpdc.2022.07.009},
issn = {0743-7315},
year = {2022},
date = {2022-07-21},
urldate = {2022-07-21},
journal = {Journal of Parallel and Distributed Computing},
abstract = {As data privacy becomes increasingly important, federated learning applied to the training of deep learning models while ensuring the data privacy of devices is entering the spotlight. Federated learning makes it possible to process all data at once while processing data independently from various devices without collecting distributed local data in a central server. However, there are still challenges to overcome for the system of devices in federated learning such as communication overheads and the heterogeneity of the system. In this paper, we propose the Adjusting Mini-Batch and Local Epoch (AMBLE) approach, which adaptively adjusts the local mini-batch and local epoch size for heterogeneous devices in federated learning and updates the parameters synchronously. With AMBLE, we enhance the computational efficiency by removing stragglers and scaling the local learning rate to improve the model convergence rate and accuracy. We verify that federated learning with AMBLE is a stably trained model with a faster convergence speed and higher accuracy than FedAvg and adaptive batch size scheme for both identically and independently distributed (IID) and non-IID cases.},
keywords = {},
pubstate = {published},
tppubtype = {article}
}
As data privacy becomes increasingly important, federated learning applied to the training of deep learning models while ensuring the data privacy of devices is entering the spotlight. Federated learning makes it possible to process all data at once while processing data independently from various devices without collecting distributed local data in a central server. However, there are still challenges to overcome for the system of devices in federated learning such as communication overheads and the heterogeneity of the system. In this paper, we propose the Adjusting Mini-Batch and Local Epoch (AMBLE) approach, which adaptively adjusts the local mini-batch and local epoch size for heterogeneous devices in federated learning and updates the parameters synchronously. With AMBLE, we enhance the computational efficiency by removing stragglers and scaling the local learning rate to improve the model convergence rate and accuracy. We verify that federated learning with AMBLE is a stably trained model with a faster convergence speed and higher accuracy than FedAvg and adaptive batch size scheme for both identically and independently distributed (IID) and non-IID cases.
2020
2.
박주원,; 김태하,; 오상윤,
HDFS 이기종 스토리지 전략을 위한 스몰파일 아카이빙🇰🇷 DomesticConference
한국차세대컴퓨팅학회 하계학술대회 MR-IoT 융합 재난대응 인공지능 응용기술 워크샵, 2020.
@conference{박주원2020HDFS,
title = {HDFS 이기종 스토리지 전략을 위한 스몰파일 아카이빙},
author = {박주원 and 김태하 and 오상윤},
url = {https://www.youtube.com/watch?v=xDjfVcu4Txw},
year = {2020},
date = {2020-12-08},
urldate = {2020-01-01},
booktitle = {한국차세대컴퓨팅학회 하계학술대회 MR-IoT 융합 재난대응 인공지능 응용기술 워크샵},
keywords = {},
pubstate = {published},
tppubtype = {conference}
}
2019
1.
박주원,; 박규동,; 오상윤,
토픽 기반 출판/구독 시스템에서 메시지 전송량 감소를 위한 k-way 메시지 통합 기법🇰🇷 DomesticConference
추계워크숍 한국정보과학회 국방소프트웨어연구회, 2019.
@conference{박주원2019토픽,
title = {토픽 기반 출판/구독 시스템에서 메시지 전송량 감소를 위한 k-way 메시지 통합 기법},
author = {박주원 and 박규동 and 오상윤},
year = {2019},
date = {2019-01-01},
urldate = {2019-01-01},
booktitle = {추계워크숍 한국정보과학회 국방소프트웨어연구회},
keywords = {},
pubstate = {published},
tppubtype = {conference}
}